Organizations have identified Artificial Intelligence (AI) as a quintessential enabling layer in their digital transformations. As AI adoption increases, so too does concern for user data privacy, coupled with the need for an agency to safeguard it. Recently, governments around the world have introduced guidelines, regulations, and bills such as the GDPR and the CCPA to improve data privacy and governance. These guidelines have explicit rules regarding the storage, processing, and use of data for any purpose, and any violation is heavily penalized.
Into this breach steps federated learning, a method of training machine-learning models that keeps user data in its location, and hence safe and private. With its privacy-by-design methodology, federated learning presents immense potential and use across data-critical industries and use cases.
Everest Group’s federated learning report defines federated learning and explores its key characteristics; compares federated learning and centralized learning; details federated learning’s benefits and applications; provides an overview of key federated learning providers; and introduces a decision-making framework for federated learning adoption.
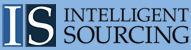